Diagnosis is a process that involves collecting data about one’s illness (collate their symptoms) and determine their anatomic, physiologic and/or psychosocial derangement (usually called disease) through clinical reasoning in order to direct a clinician toward offering specific treatments (Reed, 1992; Balogh et al, 2015).
Systems of Clinical Reasoning in the Diagnostic Process: There are two main systems of clinical reasoning used in diagnosis (Balogh et al, 2015). The first, known as the non-analytical system, relies on intuitive, automatic, and implicit processing. This system functions automatically with minimal use of working memory and is often triggered by stimuli, depending on overlearned associations or implicit learning. Examples include diagnosing congenital club foot in children during clinical examination through simple observation, or making decisions based on heuristics, i.e., recognizing a movement system diagnosis of insufficient scapular posterior tilt associated with coordination/control deficit for an individual with excessive scapular tilt during shoulder flexion (Ludewig et al, 2022). The second system involves a slower, more reflective, and analytical approach. This method requires significant working memory capacity as it involves generating mental models to hypothesize about potential outcomes or explore different scenarios, such as diagnosing lumbar radiculopathy secondary to disc herniation in patients having low back pain with referred pain in the lower extremities.
Within these two systems of clinical reasoning, there are four primary methods of diagnosis:
- Gestalt/Pattern recognition method: This method involves the immediate recognition of a patient’s presentation based on the clinician’s past experience with similar patterns of disease. It aligns closely with the non-analytical system, as it relies on intuitive recognition without extensive deliberation.
- Exhaustive evaluation method: This approach requires collecting all relevant medical information about a patient through detailed subjective questioning, physical examination, and clinical testing. It is more aligned with the analytical system, as it involves systematically evaluating all available data to reach a final diagnosis.
- Algorithm method: This method involves asking a broad, relevant question and then following pre-determined logical or evidence-based steps to reach a diagnosis. It combines elements of both systems, using intuitive prompts to guide a structured, step-by-step analytical process.
- Hypothetico-deductive method: In this method, multiple hypotheses (possible diagnoses) are formulated, and relevant clinical tests are conducted to confirm or rule out each possibility (Evans and Kakas, 1992). This method is primarily analytical, requiring significant working memory to consider and test various potential diagnoses systematically.
By understanding these systems of clinical reasoning and methods of diagnosis, clinicians can more effectively integrate intuitive and analytical approaches to enhance diagnostic accuracy and patient care.
Understanding the Hypothetico-Deductive Method
The hypothetico-deductive method in medicine involves generating diagnostic hypotheses from the initial patient information and iteratively testing these hypotheses to refine or refute them. This process begins with data collection, such as the patient’s symptoms, history, and preliminary examinations, which guides the clinician to consider several potential diagnoses. Further investigations, such as physical examinations and diagnostic tests, are then used to assess the likelihood of these potential diagnoses (Elstein & Schwartz, 2002).
The “Symptom Guided – Probability Driven” Model
The “symptom guided – probability driven” model is an effective framework for the hypothetico-deductive approach in diagnosis. This model comprises two main components:
- Symptom Guided: This aspect involves gathering initial data from the patient’s reported symptoms, medical history, and physical examination findings. These symptoms and signs serve as the primary guide for generating initial diagnostic hypotheses. According to studies, early symptom evaluation is crucial for forming a broad differential diagnosis (Graber, 2003).
- Probability Driven: After forming initial hypotheses based on symptoms, the clinician estimates the probability of each potential diagnosis. This step is informed by clinical knowledge, prevalence rates, risk factors, and evidence-based guidelines. Such ‘probabilistic thinking’ allows the clinicians to select appropriate diagnostic tests or procedures to refine these probabilities, effectively narrowing the differential diagnosis to the most likely condition (Sox et al., 2013).
Evidence Supporting the Model
The “symptom guided – probability driven” model is grounded in evidence-based practice, aligning with how clinicians often approach diagnosis in real-world settings. A systematic review by Norman et al. (2017) found that clinicians predominantly use symptom presentation to develop a list of potential diagnoses and then rely on probability estimates to guide further diagnostic testing. This method reflects an iterative process where each step refines the clinician’s understanding of the patient’s condition.
Furthermore, research on diagnostic reasoning has demonstrated the importance of probability estimation in decision-making. A study by Croskerry (2009) highlighted that diagnostic accuracy improves when clinicians use probabilistic reasoning to evaluate hypotheses, as it helps prioritize the most likely diagnoses and reduce the chances of cognitive biases, such as premature closure or anchoring.
Stages of symptom guided – probability driven’ model
There are four stages in the “symptom guided – probability driven” model, which effectively integrates the probabilistic thinking with the hypothetico-deductive method of diagnosis (Figure 1).
Stage I: In the first stage, the symptoms reported by patients are collated to form a hypothesis. All relevant clinical data collected during subjective and objective assessment is used in this stage to support making a hypothesis. Often, a single symptom (or a cluster of symptoms) can bring forth multiple hypotheses.
Stage II: In the second stage, the list of hypotheses (i.e., potential diagnoses) are ranked based on success probability. A potential diagnosis that has the highest probability of becoming true/success is ranked as first. Such prioritization is best done by considering the strength of association between the symptoms/relevant clinical data and the hypotheses.
Stage III: In the third stage, a focused subjective and objective examination is conducted to rule out the potential diagnoses that are less likely to be true.
Stage IV: In the fourth stage, a highly sensitive (most accurate) diagnostic test is performed to confirm the final diagnosis.
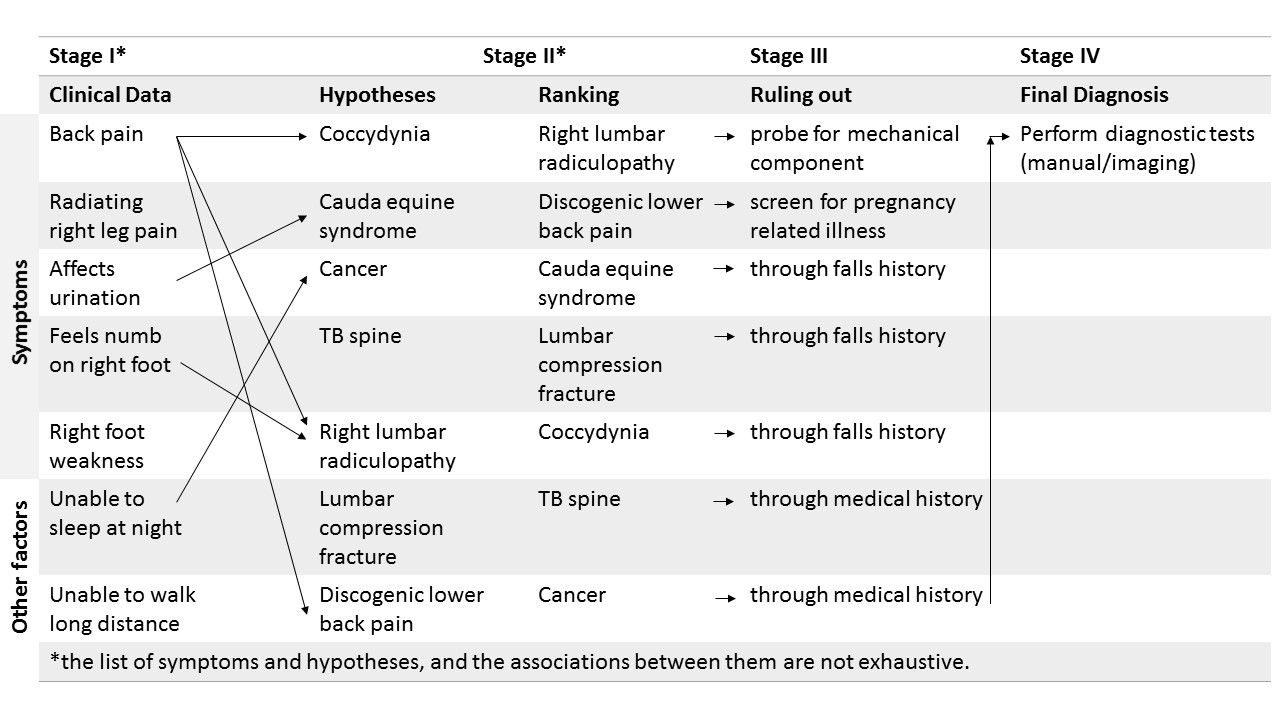
Application in Clinical Practice: A Low Back Pain Example
In clinical practice, the hypothetico-deductive method, supported by the “symptom guided – probability driven” model, helps ensure systematic and thorough diagnostic evaluation. This model involves using a patient’s presenting symptoms to guide the initial diagnostic process, followed by the application of probabilistic thinking to refine these potential diagnoses.
For example, in cases of chest pain, a common yet potentially serious symptom, clinicians use this approach to differentiate between various conditions, such as myocardial infarction, pulmonary embolism, and musculoskeletal pain. Initial hypotheses are formed based on symptom characteristics and patient history, and subsequent investigations are guided by the probability of each condition (Panju et al., 1998).
Let’s explore this approach using low back pain, a common clinical presentation, as an example (Figure 1).
Step-by-Step Application of the Model
Stage I: Symptom Guided: Generating Initial Hypotheses
When a patient presents with low back pain, the clinician begins by collecting detailed information about the symptoms. This includes the onset, duration, character, and intensity of the pain, as well as any associated symptoms such as leg pain, numbness, or weakness. The patient’s medical history, occupation, lifestyle, and any prior episodes of back pain are also considered.
For example, if a patient describes sudden onset of sharp, localized pain in the lower back after lifting a heavy object, the clinician might initially consider diagnoses such as muscle strain, ligament sprain, or herniated disc. If the pain radiates down one leg and is accompanied by numbness or weakness, the clinician might add lumbar radiculopathy (commonly caused by a herniated disc) to the list of potential diagnoses.
Stage II:. Probability Driven: Estimating the Likelihood of Each Diagnosis (Ranking)
Once initial hypotheses are formed based on symptoms, the clinician uses probabilistic thinking to estimate the likelihood of each potential diagnosis. The list of potential diagnoses are then ranked based on success probability. This step involves integrating the patient’s symptoms with clinical knowledge, prevalence data, and risk factors.
– Muscle Strain or Ligament Sprain: These are among the most common causes of acute low back pain, especially after activities that strain the back muscles or ligaments. The prevalence of these conditions in similar clinical presentations is high, making them likely diagnoses initially.
– Lumbar Radiculopathy: This diagnosis is considered when pain radiates down the leg and is associated with neurological symptoms such as numbness or weakness. The probability of lumbar radiculopathy increases if the patient’s pain follows a dermatomal pattern and if there are positive findings on the physical examination, such as a positive straight leg raise test.
– Herniated Disc: This is a common cause of lumbar radiculopathy, particularly in younger patients with a history of heavy lifting or twisting movements. The probability of a herniated disc increases if imaging studies (like MRI) reveal disc herniation compressing a nerve root correlating with the patient’s symptoms.
– Other Diagnoses: Depending on the patient’s history and presentation, other diagnoses such as spinal stenosis, spondylolisthesis, infection, or malignancy might also be considered. The probability of these conditions might be lower, but they remain part of the differential, especially if red flag symptoms (e.g., unexplained weight loss, fever, or significant trauma) are present.
Stage III: Refining Hypotheses Through Testing and Further Inquiry (Ruling out)
The next step involves gathering additional information to refine the differential diagnosis and confirm the most probable condition. This might include physical examinations, such as checking for tenderness, range of motion, reflexes, and strength testing, or ordering diagnostic tests like X-rays, MRI, or CT scans.
For example, if a patient’s symptoms strongly suggest lumbar radiculopathy, an MRI may be performed to confirm the presence of a herniated disc or nerve root compression. If imaging confirms this finding, the probability of lumbar radiculopathy caused by a herniated disc becomes very high, and the diagnosis is likely confirmed.
Stage IV: Making a Diagnosis and Formulating a Treatment Plan
Once sufficient evidence is gathered and the most likely diagnosis is identified, the clinician can make a definitive diagnosis. In this example, if the clinical examination and imaging support a diagnosis of lumbar radiculopathy due to a herniated disc, the clinician would proceed with an appropriate treatment plan, which might include pain management, physical therapy, or possibly referral to a specialist for further evaluation.
Therefore, the “symptom guided – probability driven” model provides a structured approach to diagnosing low back pain by using symptoms to guide the formation of initial diagnostic hypotheses and applying probabilistic thinking to test and refine these hypotheses. This iterative process ensures a thorough and clinical reasoning-based approach to diagnosis, ultimately improving patient outcomes.
To further appreciate how the “symptom guided – probability driven” model provides a robust framework for applying the hypothetico-deductive method of diagnosis in practice, it is necessary to understand the meaning, advantages, and disadvantages of probabilistic thinking.
What is Probabilistic Thinking? Probabilistic thinking in diagnosis involves assessing the likelihood of different potential diagnoses based on available data, clinical evidence, and the prevalence of conditions. This approach is central to the “symptom guided – probability driven” model and the hypothetico-deductive method. Like any diagnostic approach, probabilistic thinking has its advantages and disadvantages.
Advantages of Probabilistic Thinking in Diagnosis
1. Improved Diagnostic Accuracy:
– Integration of Evidence-Based Medicine: Probabilistic thinking allows clinicians to integrate evidence-based guidelines and statistical data into their decision-making process, improving diagnostic accuracy. By considering the prevalence and likelihood of various conditions, clinicians can prioritize common and likely diagnoses, reducing the chances of missing common conditions.
– Reduces Cognitive Biases: By focusing on probabilities rather than subjective impressions, probabilistic thinking helps reduce cognitive biases such as anchoring (relying too heavily on the first piece of information encountered) and availability bias (overestimating the likelihood of events that are more memorable). This approach encourages a systematic evaluation of all possible diagnoses based on their likelihood.
2. Efficient Use of Resources:
– Guided Diagnostic Testing: By estimating the probability of different diagnoses, clinicians can prioritize diagnostic tests and procedures that are more likely to yield useful results. This targeted approach helps avoid unnecessary tests and reduces healthcare costs and patient burden.
– Streamlined Decision-Making: Probabilistic thinking can streamline the decision-making process by allowing clinicians to focus on the most likely diagnoses, reducing the time spent on less probable conditions. This efficiency is especially beneficial in high-pressure environments like emergency departments.
3. Improved Patient Communication and Shared Decision-Making:
– Transparent Risk Communication: Using probabilities allows clinicians to effectively communicate risks and uncertainties to patients. This transparency can help patients understand the rationale behind diagnostic and treatment decisions, fostering trust and collaboration.
– Facilitates Shared Decision-Making: Probabilistic thinking encourages shared decision-making by involving patients in discussions about the likelihood of various conditions and the potential benefits and risks of different diagnostic tests or treatments.
Disadvantages of Probabilistic Thinking in Diagnosis
1. Complexity and Cognitive Load:
– Cognitive Overload: The process of constantly updating probabilities based on new data can be cognitively demanding for clinicians. This can lead to errors, especially in fast-paced clinical settings where time and mental resources are limited.
– Requires Advanced Training and Understanding: Probabilistic thinking often requires a good understanding of statistics and the ability to interpret data critically. Not all clinicians may have the necessary training or experience to apply probabilistic reasoning effectively, potentially leading to incorrect assessments.
2. Potential for Over-Reliance on Statistics:
– Neglect of Clinical Judgment: While probabilities provide valuable guidance, they should not replace clinical judgment. An over-reliance on statistical probabilities may cause clinicians to overlook unique aspects of a patient’s presentation or rare conditions that are not statistically likely but still possible.
– Challenges with Atypical Presentations: Probabilistic thinking is often based on typical presentations of diseases. Atypical cases or rare conditions might be underappreciated or missed if the focus is too heavily on the most likely diagnosis based on probabilities.
3. Difficulty with Limited Data:
– Inaccuracy with Insufficient Information: Probabilistic reasoning relies heavily on the availability of accurate data. When data is limited or of poor quality, the probabilities calculated may be inaccurate, leading to potential misdiagnoses.
– Variability in Data Sources: Different studies and guidelines may provide varying probability estimates for the same condition. This variability can lead to confusion and inconsistency in applying probabilistic reasoning, particularly in cases where guidelines are not up-to-date or are conflicting.
4. Patient Misunderstanding and Anxiety:
– Complexity in Communication: While probabilistic thinking can aid in patient communication, it can also create confusion if not conveyed clearly. Patients may have difficulty understanding probabilistic information, especially if it involves complex statistical concepts.
– Potential for Increased Anxiety: Discussing probabilities and risks may sometimes increase patient anxiety, particularly if the probability of a serious condition is low but not negligible. This can lead to patient worry and pressure for additional, possibly unnecessary, testing.
Therefore, probabilistic thinking offers significant advantages in diagnostic accuracy, efficiency, and patient communication by systematically evaluating the likelihood of various conditions. However, it also presents challenges, including cognitive demands, potential over-reliance on statistics, difficulties with limited data, and complexities in patient communication. Balancing probabilistic reasoning with clinical judgment and individual patient context is essential for optimizing diagnostic outcomes. As diagnostic medicine continues to evolve, incorporating probabilistic thinking and evidence-based practice remains essential for improving diagnostic accuracy and patient outcomes.
Reference:
- Reed, R.L., 1992. Clinical Epidemiology: A Basic Science for Clinical Medicine. The Journal of the American Board of Family Practice, 5(6), pp.660-660.
- Committee on Diagnostic Error in Health Care; Board on Health Care Services; Institute of Medicine; The National Academies of Sciences, Engineering, and Medicine; Balogh EP, Miller BT, Ball JR, editors. Improving Diagnosis in Health Care. Washington (DC): National Academies Press (US); 2015 Dec 29. 2, The Diagnostic Process. Available from: https://www.ncbi.nlm.nih.gov/books/NBK338593/
- Ludewig PM, Saini G, Hellem A, et al. Changing our Diagnostic Paradigm Part II: Movement System Diagnostic Classification. IJSPT. 2022;17(1):7-17. doi:10.26603/001c.30177
- Evans, C. and Kakas, A.C., 1992. Hypothetico-deductive Reasoning. In FGCS (pp. 546-554).
- Croskerry, P. (2009). A universal model of diagnostic reasoning. Academic Medicine, 84(8), 1022-1028.
- Elstein, A. S., & Schwartz, A. (2002). Clinical problem solving and diagnostic decision making: selective review of the cognitive literature. BMJ, 324(7339), 729-732.
- Graber, M. L. (2003). Diagnostic error in internal medicine. Archives of Internal Medicine, 163(20), 2499-2504.
- Norman, G. R., Monteiro, S. D., Sherbino, J., Ilgen, J. S., Schmidt, H. G., & Mamede, S. (2017). The causes of errors in clinical reasoning: Cognitive biases, knowledge deficits, and dual process thinking. Academic Medicine, 92(1), 23-30.
- Panju, A. A., Hemmelgarn, B. R., Guyatt, G. H., & Simel, D. L. (1998). The rational clinical examination. Is this patient having a myocardial infarction? JAMA, 280(14), 1256-1263.
- Sox, H. C., Higgins, M. C., & Owens, D. K. (2013). Medical Decision Making. John Wiley & Sons.